Let’s take the following statement:
“Highly intelligent women tend to marry men who are less intelligent than them.”
I often use an outrageous statement like this at parties. “You know I came across this interesting study which found that high IQ women tend to marry men who are somewhat less intelligent than they are. It’s fascinating!” I can get a good conversation started by asking for an explanation, to which people readily oblige.
Whenever we try to explain such statements, we spontaneously ask ourselves what might be the possible reason for this to happen, i.e, what was the “cause” for this “effect”.
The popular reasons I usually hear are: highly intelligent men don’t want an intelligent wife to steal their thunder, hence the intelligent women have to settle for less intelligent men. Or, high IQ women want to avoid competition of equally intelligent men (which is exactly the opposite of the previous argument). Or, my favourite: unlike the low IQ men, high IQ men aren’t charmers.
If you try this at a party, I’m sure you’ll come across more far-fetched explanations. It’s good fun. Now consider this statement:
“The correlation between the intelligent scores of spouses is less than perfect.”
This is a (mathematical and) boring statement. But it’s a true statement. Interestingly, this lacklustre statement and the above (interesting) statement point towards more or less the same thing. Before I explain that, let’s understand what’s correlation. A slight detour:
If you take a large number of random earthlings (say ~1,000,000) from all age groups, genders, races, cultures, etc., and plot the relationship between their height and weight, the graph would look something like this.
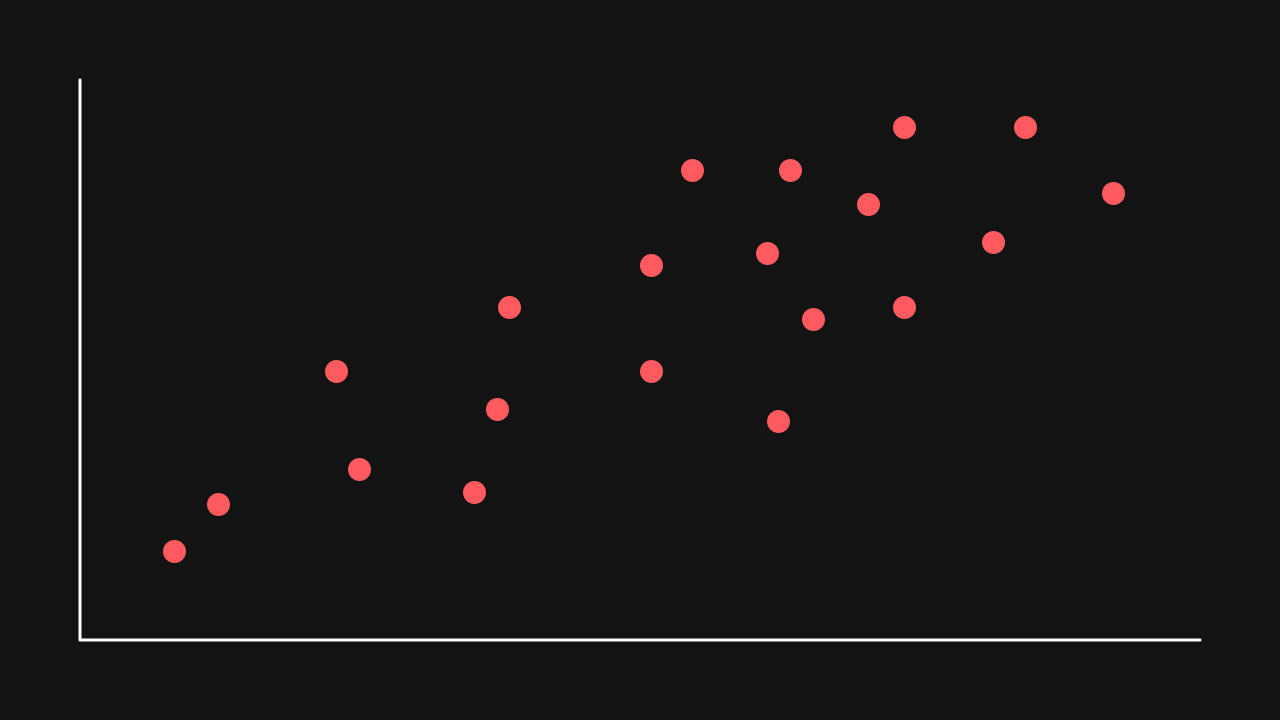
While there is some pattern, we cannot find a “perfect relationship” between the height and weight of a person. While virtually every three-year-old will be lighter and shorter than every grown man, not all grown men or three-year-olds of the same height will weigh the same. In technical terms, the correlation coefficient between height and weight is less than 1 (not perfect) but not 0 (i.e., there is some correlation).
But if we take another example where we plot the number of cricket matches India wins every year against the total Coca-Cola consumption in the country, the graph would be all over the place. There is no correlation, i.e., the correlation coefficient is zero.
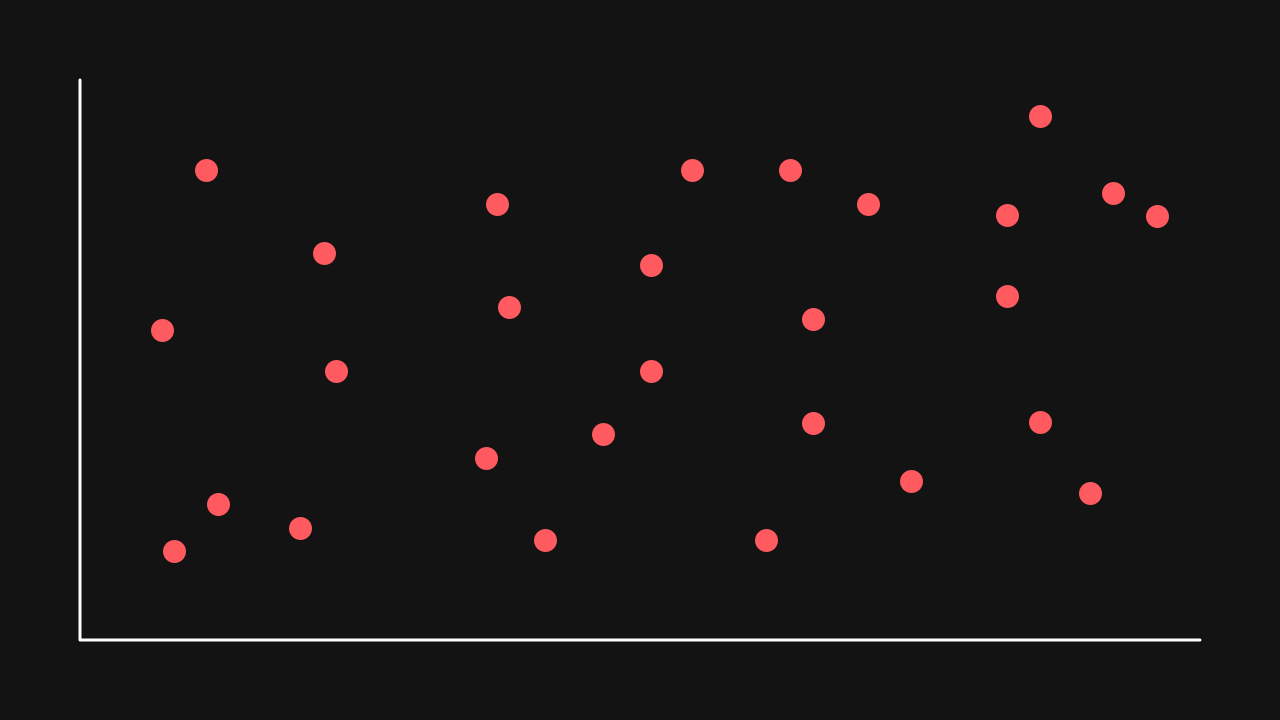
The third use case, where two entities have perfect correlation (i.e., correlation coefficient is either 1 or -1), is often pretty obvious. For example, the relationship between Celsius value and Fahrenheit value of temperature. If you plot them on a graph, you’ll get a straight line.
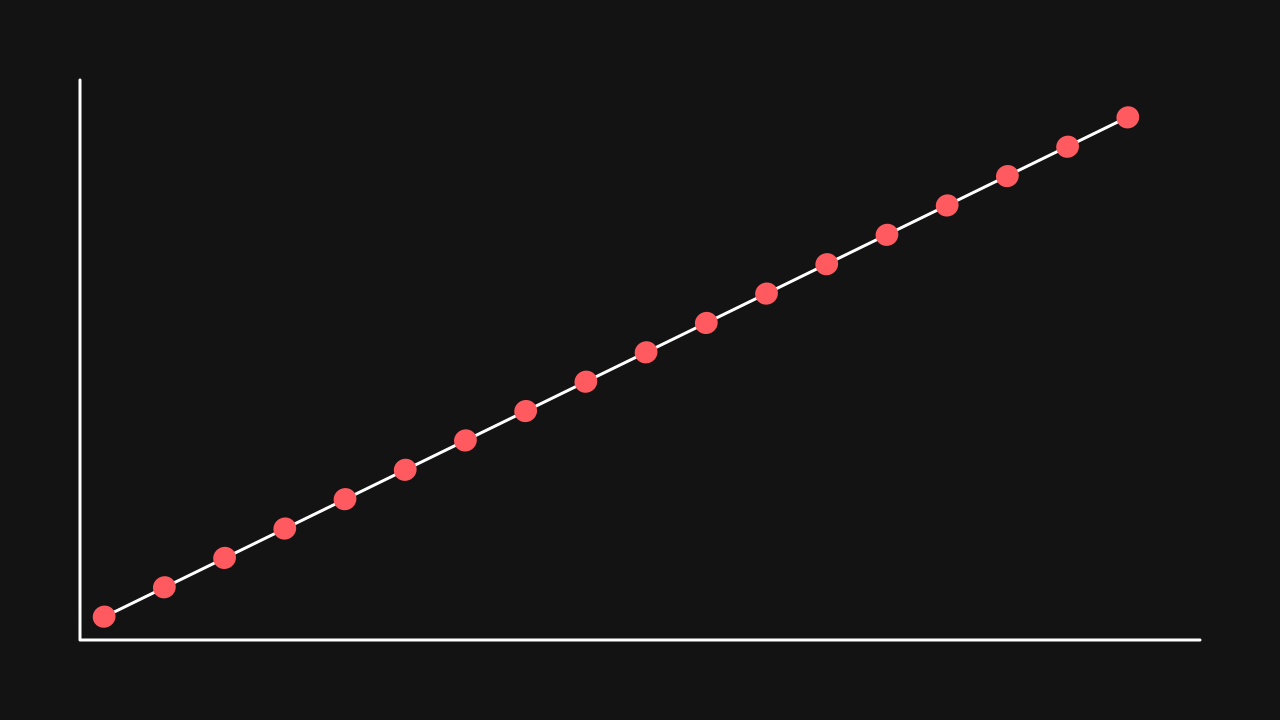
Similarly, an example of a negative correlation (correlation coefficient of -1) is the relationship between speed of a vehicle and the time it takes to reach from point A to B. If speed increased, time decreases (given everything else remains the same).
Now, coming back to the case at hand. On the basis of what we’ve learnt about correlation, the statement, “The correlation between the intelligent scores of spouses is less than perfect,” means: if we take a large enough sample of couples and plot the relationship between their individual IQs on a graph, we might see some pattern (positive/negative correlation), but we won’t get a straight line (i.e., they have less than perfect correlation).
In other words, if the correlation between the IQ of spouses is less than perfect and if we believe men and women do not differ in intelligence, then it is a mathematical inevitability that highly intelligent women will be married to men who are on average less intelligent than they are—and vice versa. Meaning, even high IQ men tend to marry women who are less intelligent than they are.
This essentially means that the chances of two partners representing the top 1% in terms of intelligence (or as a matter of fact, any characteristics whatsoever) is far smaller than one partner representing the top 1% and the other the bottom 99%—thereby bringing down their combined intelligence towards 50% (or, towards the mean). This very phenomenon is known as Regression Towards the Mean.
Regression towards the mean is what explains why high IQ people marry people with average intelligence. It has nothing to do with culture, fear, relationship quality, stigma, social media, personality, jealousy, charm, charisma, etc. It’s a purely mathematical phenomenon—one that cannot be expressed with “reasoning”.
In fact, correlation and regression are not two concepts—they are different perspectives on the same concept. Daniel Kahneman, the father of behavioural economics, observes that whenever the correlation between two entities is imperfect, there will be regression towards the mean. That’s just how nature works.
But even after all the explanation, something feels off. Your mind is strongly biased toward causal explanations and cannot swallow the reason for something being “mere statistics.” That’s the limit of our reasoning.
Regression has an explanation, but it doesn’t have a cause. No matter what reason you attach (believable or not), it will be wrong. So, don’t bother.
Regression confounds me. The only way I tame my “reasoning urges” is by taking stock of The Green Lumber Fallacy: just because I cannot explain something, doesn’t make it less true.
Reality doesn’t rely on narratives, humans do. When you have action (and result), you don’t need explanation (of the cause and effect).
Therefore, next time someone tries to explain why ice-cream causes heatstroke, or why depressed children treated with energy drinks improve significantly over a couple of months, humour them. But don’t try to correct them unless you never want to be invited to parties ever again.